Théo Uscidda
Ph.D. with Marco Cuturi @ CREST - ENSAE, Institut Polytechnique de Paris
Visiting Ph.D. hosted by Fabian Theis @ Helmholtz Munich, Technical University of Munich
Internships: incoming (winter) @ Amazon AI, Fundamental Research Team– past @ Flatiron Institute, Simons Foundation.
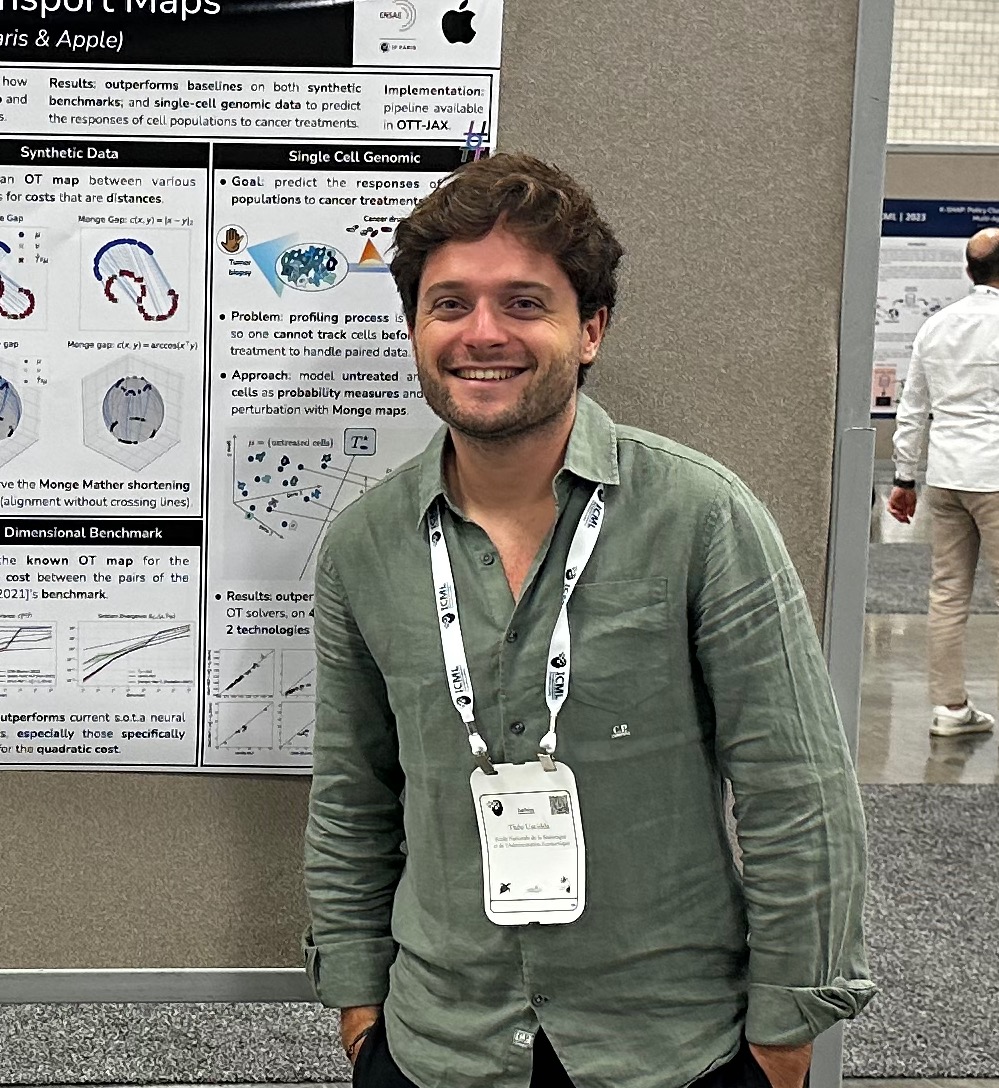
Education: I am a Ph.D. student in machine learning working with Marco Cuturi (Apple MLR). Prior to that, I completed a master’s degree in Mathematics, Vision and Learning (MVA) at ENS Paris-Saclay. During my master’s thesis, I worked with Claire Boyer (Sorbonne Université), Julie Josse (INRIA) and Boris Muzellec (Owkin) at LPSM.
Research: I work on the interplay between optimal transport (OT), generative modeling, and representational learning. I intend to demonstrate how OT can introduce optimality structures within flow-, diffusion-, and VAE-based models, enhancing performance. I have applied these methods across diverse areas, including image generation/unpaired image translation, disentangled representation learning, optimization over probability spaces, geographic information, and various single-cell genomics challenges, such as reconstructing cellular trajectories, predicting cellular responses to perturbations, and translating between different single-cell data modalities. Recently, my focus has been on using OT to enhance/analyze the properties of vision/language foundation models.
Experiences: Since February 2024, I have been a visting Ph.D. student in Fabian Theis’ lab at Helmholtz Munich, where I have been working on generative models for single-cell perturbation discovery. Additionally, from June to August 2024, I interned at the Flatiron Institute collaborating with Victor Chardes and Surya Maddu in Michael Shelley’s team to develop generative models for inferring biophysical dynamics from omics data. During winter 2024, I will be interning with the fundamental research team of Amazon AI with Matthew Tragger and Alessandro Achille, where I will work on large language models.
news
Sep 26, 2024 | Thrilled to announce two accepted papers at NeurIPS 2024: GENOT: Entropic (Gromov) Wasserstein Flow Matching and Mirror and Preconditioned Gradient Descent in Wasserstein Space, with the latter receiving a spotlight! Huge thanks to my coauthors for these amazing collaborations. See you in Vancouver! |
---|---|
Sep 2, 2024 | Back in Munich! I have rejoined Fabian Theis’ lab at Helmholtz Munich, where I will keep working on single-cell perturbations. |
Jun 20, 2024 | I have joined the Flatiron Institute, where I will spend the summer working with Victor Chardes and Surya Maddu, within Michael Shelley’s team. I will be developing generative models to infer biophysical dynamics from omics data. See you in New York City! |
Apr 25, 2024 | I presented the unbalanced Monge maps at Google DeepMind’s reading group on generative models, transport and sampling, organized by Valentin de Bortoli and Arnaud doucet. Looking forward to presenting the paper at ICLR 2024! |
Feb 1, 2024 | I have joined Fabian Theis’ lab at Helmholtz Munich, where I will be developping generative models for discovering single-cell perturbations. |